Development and discovery of new materials using Materials Informatics (MI)
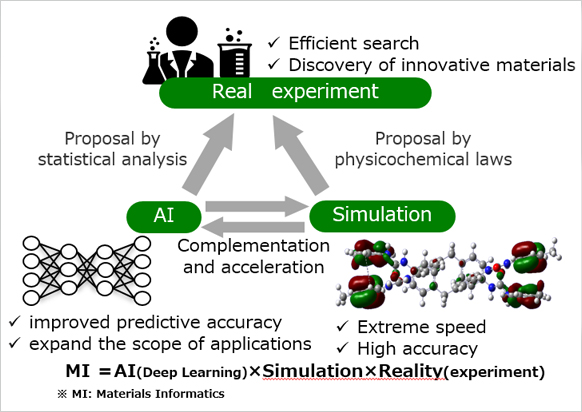
Materials Informatics (MI) is a promising field of study where vast amounts of materials data are processed by AI and deep learning. Although materials R&D activities have long been carried based on extensive experiments and researchers' intuition, the scope of MI applications is rapidly expanding.
ENEOS' goal is to discover and develop innovative materials for a sustainable low carbon future in the fields of renewable energies, catalysts, functional materials and lubricants. By integrating experiments, simulations and AI, ENEOS aim to realize materials development through "physicochemical laws" and "statistical analysis".
AI x Simulation Platform: Matlantis™
Recent advances in simulations and AI technologies drastically accelerate materials R&D. In-silico atomistic-scale simulations predicting materials properties are suitable for MI to generate and analyze huge amount of materials data.
ENEOS have developed the universal atomic-level simulator Matlantis™ in collaboration with Preferred Networks (PFN). In 2021, these two companies established a joint-venture called Preferred Computational Chemistry (PFCC) to offer Matlantis™ as a SaaS solution. Matlantis™ computes materials properties at extreme speed with high accuracy. It can predict the structures and properties of molecules, crystals, amorphous structures, interfaces and even macromolecules.
ENEOS are also focusing research efforts on low carbon energy carriers such as hydrogen, accelerating the pace of materials R&D with Matlantis™.
Case Study 1: Virtual Screening of Catalysts for Ammonia Synthesis
To enhance catalyst design strategy, reaction efficiencies of several alloy catalysts were calculated using Matlantis™ and the results analyzed by means of reaction rate simulations. Candidate materials exhibiting high reaction efficiencies were discovered within a week, while similar calculations would have taken years using conventional simulation tools.


Case Study 2:Design of Lubricants and Greases
With Matlantis™, ENEOS design lubricants and greases to improve the efficiency and performance of mechanical systems and reduce their environmental impact. For instance, by identifying the molecular structures of additives that enhance friction and wear properties or by clarifying chemical reactions that are crucial for wear resistance through the elucidation of complex phenomena. Based on these simulation results, ENEOS are promoting the design of ideal lubricants and greases that can achieve the desired tribological properties.


Case Study 3: Multi-Scale Simulation×AI for Tire Rubber Material Development
ENEOS employ Matlantis™ and other simulation tools to develop rubber materials for tires with complex hierarchical structures. Finite Element Methods (FEM) and molecular dynamics (MD) are conventionally used to develop rubber materials, but Matlantis™ has further expanded the computational domain to the atomic scale. The combination of these simulation techniques at various scales with machine learning (AI) is driving the development of materials that contribute to improved tire performance.
